It is said that imitation is the sincerest form of flattery. For certain weeds, it can mean survival.
Researchers have found that different weed species will evolve to look similar to the crop in which they are found, a phenomenon called weed mimicry. When weeds mimic their visual appearance to match a crop, they can be left to grow and multiply, or may even trick a hand weeder into terminating the crop plant instead.
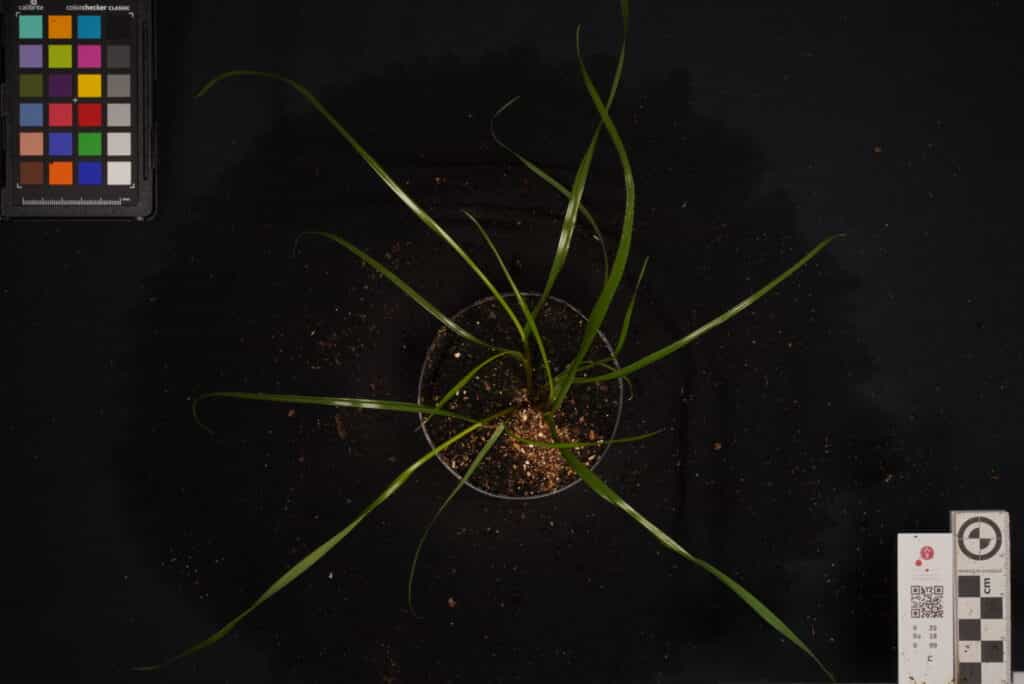
Guy Coleman, a post-doctoral researcher at the University of Copenhagen in Denmark, is working with a team to understand how image-based weed recognition for site-specific weed management technology could also lead to weeds evolving to look like the crop they invade. After all, this technology uses electronic eyes, or cameras, mounted on a sprayer boom or a drone, to visually identify weeds and then directly spray them with herbicides.
Could weeds use the old, tried-and-true practice of mimicry to dodge our newest weed management technology?
Coleman, who recently submitted his doctorate in precision agriculture and site-specific weed control, is using the challenging combination of wheat and annual ryegrass to help identify if ryegrass will evolve mimicry to the wheat. There are other crop–weed combinations where mimicry has evolved, including rice and barnyardgrass, sorghum and johnsongrass, corn and Mexican teosinte (Zea mexicana).
“We are conducting a series of greenhouse selection experiments for crop mimicry using an image-based algorithm,” says Coleman. “The algorithm we’re working with is currently trained to classify a plant into either wheat or annual ryegrass.” His team is at the preliminary stages of this work, crossing these ryegrass plants and watching to understand how the physical traits of each population change after image-based selection.
In a recently published journal paper, Coleman and the team explain the details of crop mimicry, which includes three components: the model (or crop) being imitated, the mimic (or weed) that imitates the crop, and the agent, or “dupe” as he calls it, that is fooled by the mimic. The dupe could be the human eye or the image-based weed termination technology. When the dupe can’t tell the crop and weed apart, the mimic wins the battle and its genetics are passed to the next generation.
Mimics Face Off With Precision Weed Management Technology
Over time, as weeds continue their physical evolution, mimicry could become an issue, Coleman says.
“I don’t think image-based weed detection technologies will be uniquely immune from the evolution of avoidance mechanisms in weeds,” remarks Coleman. “Through hand-weeding in rice, humans drove the evolution of distinct changes in barnyardgrass. If that selection led to mimicry, why shouldn’t algorithms? What’s even more concerning is that this technology will be used over much greater areas and more frequently than what likely led to the development of crop mimicry in barnyardgrass.”
“Through hand-weeding in rice, humans drove the evolution of distinct changes in barnyardgrass. If that selection led to mimicry, why shouldn’t algorithms?”
Guy coleman
But camera or visual technology for weed identification is rapidly becoming prevalent in precision ag. Dr. Eugene Law, a weed ecologist and assistant professor at Ohio State University, explains why image-based technology may be able to overcome a weed’s talent of mimicry.
“Computers and technology have advantages,” Law explains. “Unlike humans, they don’t get too tired, bored, or hot. They don’t get visual fatigue looking at the same thing over and over. Computer vision-based precision tools can do these repetitive tasks like weeding with fewer errors.”
Of course computers are not error-immune. The most common algorithms used to detect weeds examine the plant’s visual characteristics such as leaf shape, color and texture – the same visuals humans use to decide whether it’s weed or not. But Law says that in addition to the physical characteristics, the algorithms can incorporate multiple data streams that aren’t based on what’s visible to the human eye alone. Sensors that can capture infrared or multispectral images to look at the plants on a deeper level can provide an additional layer of data to identify weed species, especially when weeds reflect various light wavelengths differently than the crop.
“Also, as we move forward developing these technologies, computer decision-making needs to be addressed when they encounter situations where it is difficult to distinguish between the crop and a weed,” Law says. “When does the computer ‘decide’ to spray or not? Does it mistakenly spray a crop plant or overlook a weed? The programmers and end-users of the technology will need to decide on which side to err.”
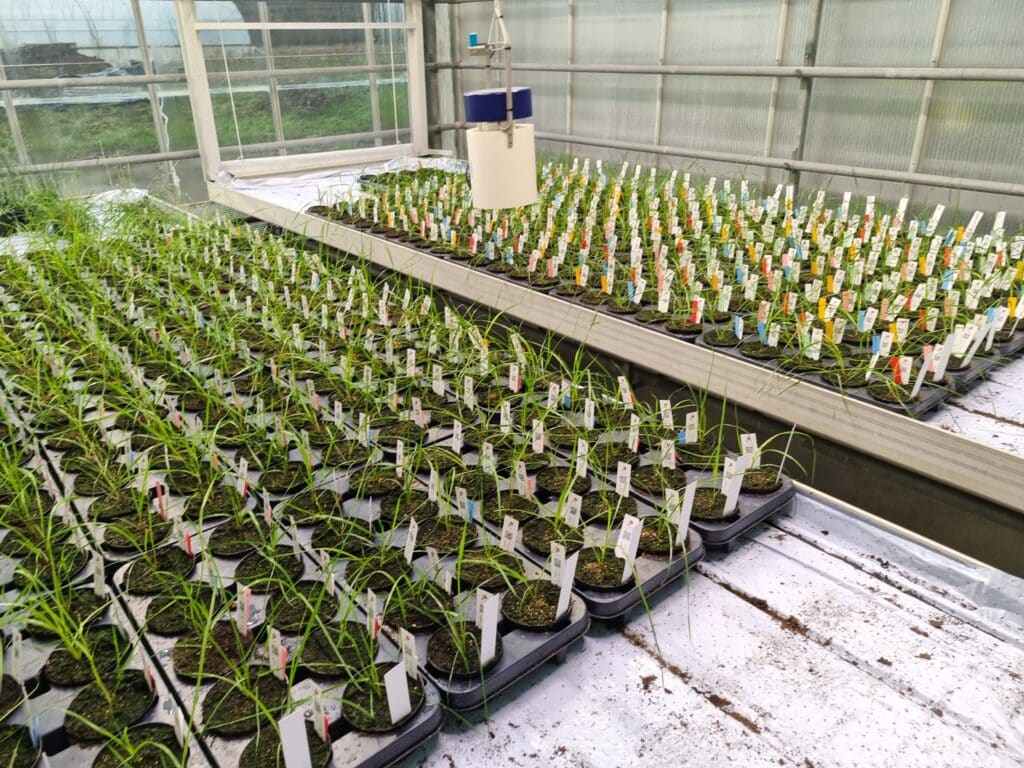
Covering All Bases
The advantages of precision spray technology include targeting weeds precisely, which uses less product across a field, saving the farmer input costs. But as cool as new technology can be, farmers need to be aware of its limitations. Relying solely on a sensor to catch a weed in a field is not fool-proof.
“Regarding all weed control tools, never rely on one thing,” Coleman says. “As with herbicides, integrated weed management will continue to be important. We need to ensure weed selection algorithms are not the only thing. If farmers rely on an algorithm to, for example, reduce weeds by using precision tillage and spraying a herbicide, the algorithm is making the selection in both instances.”
Integrated weed management includes applying different approaches to control weed populations. Using a combination of herbicides along with mechanical and cultural methods will go a long way to reduce weed issues and avoid the evolution of resistance to any one tactic.
To learn more about how individual tactics might influence common weed populations, see The Weed Management Planner.
To learn more about precision weed management and the use of image-based weed detection see GROW’s webpage on Precision Weed Management.
Article by Carol Brown; feature and header photos by Guy Coleman